Key Takeaways
1
Pattern Recognition utilizes sEMG signals as input data to recognize intended movement patterns of the user. These movement patterns are classified and can be applied to the actuation of a prosthetic, making the prosthetic obtain a higher degree of functionality.
2
Two studies looked at the effect on the actuation accuracy of prosthetics from the characteristics of the input data. The first study looked at input data type: if adding kinematic data to EMG data enhanced the pattern recognition accuracy. The second looked at the integrity of the input data: if muscle signals from unilateral congenital below-elbow deficiency (UCBED) patients can be utilized as pattern recognition input data.
3
Results showed that adding kinematic data along with EMG data as inputs increased the actuation accuracy of the prosthetic. In addition, the muscle signals of patients with UCBED can be used as input data as the muscle signals produced were detectable and the patients were observed to have some degree of control.
Pattern recognition refers to the use of surface electromyography (sEMG) to recognize human movements algorithmically. Once these movements are recognized within the program, they are translated into distinguishable motion that is carried out by a prosthetic. EMG pattern recognition helps patients regain a higher degree of functionality and usability of their prosthetic. Despite on-going research, upper-limb prosthetics with pattern recognition are often abandoned by end users due to their inconsistency and inaccurate movement performance; approximately 35%-45% of pediatric upper-limb prosthetics are discarded. Therefore, there is a great need to improve the movement accuracy of these systems, especially upper-limb prosthetics.
Whether acquired during birth, or experienced after a stroke, upper-limb deficiencies rob a patient’s ability to perform everyday tasks. Approximately 17 million people, globally, suffer from strokes every year, of which 80% experience an upper-limb motor impairment. In addition, congenital upper-limb deficiencies appear every 1 in 500 births.
Treatments for upper-limb deficiencies commonly involve upper-limb prosthetics. However, where these upper-limb prosthetics fail is the ability for patients to use their prosthetic in a realistic manner. An abundance of research has been poured into advancing these upper-limb prosthetics to autonomically move as their natural limb would. Accurate pattern recognition, driven by EMG, can further progress this research to achieve the long-term goal of realistic actuation.
Two recent studies looked to improve the accuracy of pattern recognition. Different approaches were taken by both papers. The first study, performed by Hui Zhou and his team from the Nanjing University of Science and Technology, proposed an improvement of their sEMG pattern recognition platform by pairing it with a Leap Motion controller to provide kinematic data. The second study, performed by Marcus Battraw and his team from the University of California, Davis, studied the ability of pediatric patients with unilateral congenital below-elbow deficiency (UCBED) to actuate their affected muscles.
Hui Zhou and his team focused their research on hand rehabilitation devices and hand pattern recognition. By giving the system an additional set of inputs, like kinematic data, it was hypothesized that the accuracy of average hand pattern recognition would improve in comparison to only EMG inputs. The system was tested using two methods of input implementation: feature fusion and decision fusion. Feature fusion involves extracting the EMG as well as the kinematic features and fusing them as inputs before inserting them into the classifier. Decision fusion refers to the EMG and the kinematic features being implemented into the classifier separately and their outputs being superimposed into one classification result. These fusion methods were compared, for movement accuracy, to EMG only and kinematic only inputs. Out of the four methods, the feature fusion method displayed a higher pattern recognition accuracy of 96.43%. This study showed that adding a kinematic type of data into the classifier input is beneficial for pattern recognition accuracy and can therefore provide the prosthetic with more accurate movements.
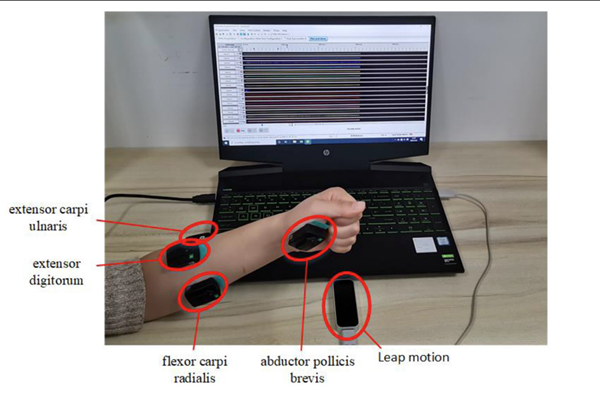
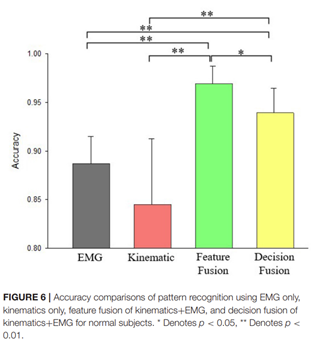
Marcus Battraw and his team researched the integrity of using muscle signals from UCBED pediatric patients as input for a pattern recognition classifier. Their protocol involved performing 10 different movements with seven sEMG electrodes adhered around both the affected and unaffected limbs. The time and frequency domains of their sEMG data were observed and compared between the two limbs. It was found that the affected sides produced detectable and consistent muscle activity. The results of the study showed that congenital one-handed pediatric patients retain some degree of control over their affected muscles. This gives confidence that advanced control prosthetics are applicable for these patients, as their muscle signals are proven to be capable of being detected, translated, and refined.
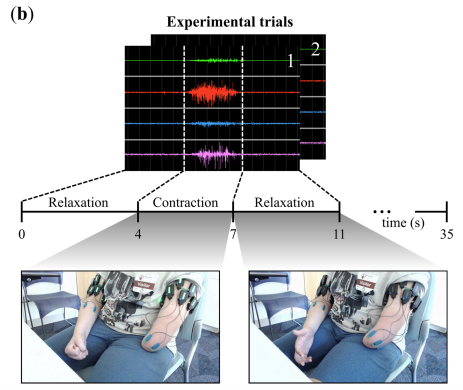
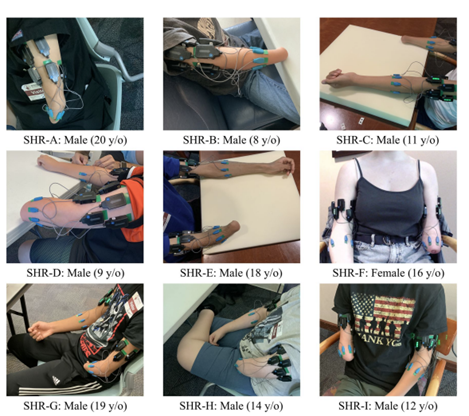
Both authors, although approached their research in contrasting ways, provided a greater understanding of what factors can influence and improve pattern recognition accuracy. In Hui Zhou’s study, the accuracy of the input data type was assessed and was found to be very influential towards outputting a more precise prosthetic actuation. In Marcus Battraw’s study, the viability of EMG input data stemming from muscle signals of UCBED patients was determined and deemed applicable for pattern recognition. These two papers showcase that while there is still a lot of research that still needs to be done to improve pattern recognition accuracy, the integrity of input data that is processed for pattern recognition is vital.
For more information on pattern recognition and the use of EMG for prosthetic control, reach out to us at contact@delsys.com and learn more about the research area on Delsys Scholar.